The Intelligent Automation Platform for Healthcare.
Hundreds of practices and healthcare service businesses use Tennr to automate their document flow and manual payor processes to drive more patient referrals, see patients faster, and reduce denials, all while growing with 1/10th the headcount.
Book a Demo
Wrangle, label, and triage every document
Tennr labels, tags, and categorizes each document passing through your fax queues and inboxes. Whether it’s a new or existing patient, additional documentation, or documents only relevant to your billing team (e.g. an EOB or a check) we make sure it gets handled appropriately.
The Machine Learning Under the Hood
Triage VLM™ classifies and tags each page within a given set of documents. Triage automatically separates the junk from important documents and informs what documents should be sent to which workflows.
Triage VLM is a proprietary self-learning Vision-Language model (VLM). VLM is a novel approach inspired by training techniques popularized in late 2023 to reason not only on the language of a document, but also on the visual layout of a document. This is especially important in healthcare, as many documents are categorized using visual indicators like reports, tables, charts, and signatures.
Because each practice tags documents differently based on per-company rulesets, Triage VLM self-learns for each Tennr customer. Each customer runs separate ‘weights’ that quickly learn, which drives accuracy to >91% after the first 100 documents and to >96% after the first five hundred.
Tennr Multi Patient™ is a very specialized language model built to identify how many patients are present in a document and on which pages each patient shows up so the document can be split by patient. Because so many documents come in that have information for many patients, it’s crucial that you can ‘split’ a document up patient by patient. Doing this across every single packet coming through a business has required an incredibly specialized and lightweight model trained solely on identifying unique individual patient identifiers.
Triage VLM is a proprietary self-learning Vision-Language model (VLM). VLM is a novel approach inspired by training techniques popularized in late 2023 to reason not only on the language of a document, but also on the visual layout of a document. This is especially important in healthcare, as many documents are categorized using visual indicators like reports, tables, charts, and signatures.
Because each practice tags documents differently based on per-company rulesets, Triage VLM self-learns for each Tennr customer. Each customer runs separate ‘weights’ that quickly learn, which drives accuracy to >91% after the first 100 documents and to >96% after the first five hundred.
Tennr Multi Patient™ is a very specialized language model built to identify how many patients are present in a document and on which pages each patient shows up so the document can be split by patient. Because so many documents come in that have information for many patients, it’s crucial that you can ‘split’ a document up patient by patient. Doing this across every single packet coming through a business has required an incredibly specialized and lightweight model trained solely on identifying unique individual patient identifiers.
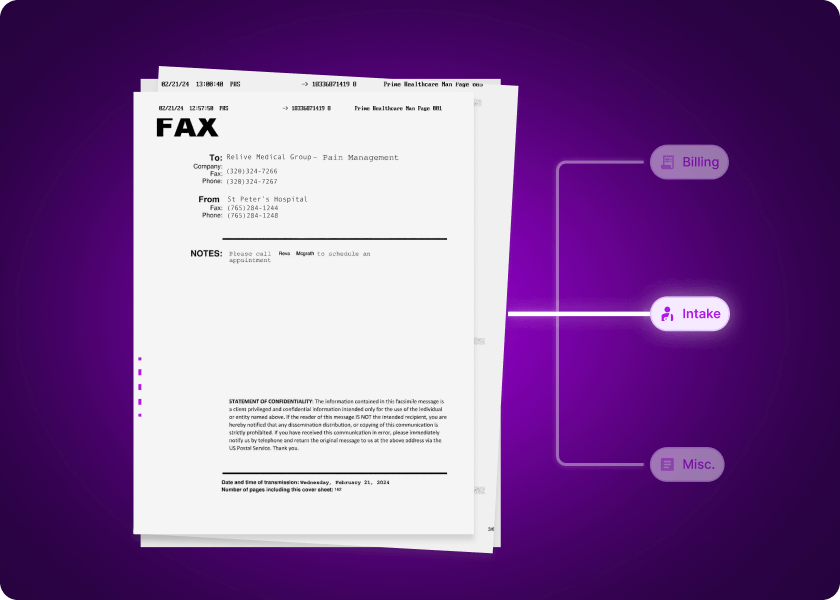
Create charts and orders without going cross-eyed
Automatically populate your EHR or billing platforms with the relevant patient and billing information quickly and accurately every time.
The Machine Learning Under the Hood
Tennr's flagship document reasoning model, RaeLM™, (named after one of our earliest users ) extracts complex medical data from messy orders, referrals, and notes.
RaeLM is a bit of a misnomer, as it’s really a chorus of models: a checkbox identification and classification model, a vision model for factoring in page, table and chart layout, and finally a language model built to decode all the above information into simple, human readable structured data.
Sidenote: Before Tennr, the highest performing checkbox reader commercially available, benchmarked at under 64% accuracy rates across random samplings of the healthcare data Tennr processes daily. RaeLM is now benchmarking above 98%. To do this, Tennr maintains the world’s largest dataset of labeled medical checkbox data pairs.
A key feature of RaeLM that we closely monitor is its confidence level. For each value RaeLM extracts, we have an accompanying ‘confidence’ interval. This number allows Tennr users to turn on ‘High Confidence Autopilot’, where work is reviewed by humans only where the model’s confidence falls below a specified threshold (typically at 98% confidence interval). With fallback only when RaeLM is ‘low-confidence’, Tennr is driving >99.8% accuracy on all data entry. In a sampling study across our broad user-base, we found the average human makes a mistake on around ~8% of all data fields, or just 92% accuracy. This number varies given time of day and individual energy levels. RaeLM has yet to show signs of a Friday slump, but we’ll report back if this changes.
RaeLM is a bit of a misnomer, as it’s really a chorus of models: a checkbox identification and classification model, a vision model for factoring in page, table and chart layout, and finally a language model built to decode all the above information into simple, human readable structured data.
Sidenote: Before Tennr, the highest performing checkbox reader commercially available, benchmarked at under 64% accuracy rates across random samplings of the healthcare data Tennr processes daily. RaeLM is now benchmarking above 98%. To do this, Tennr maintains the world’s largest dataset of labeled medical checkbox data pairs.
A key feature of RaeLM that we closely monitor is its confidence level. For each value RaeLM extracts, we have an accompanying ‘confidence’ interval. This number allows Tennr users to turn on ‘High Confidence Autopilot’, where work is reviewed by humans only where the model’s confidence falls below a specified threshold (typically at 98% confidence interval). With fallback only when RaeLM is ‘low-confidence’, Tennr is driving >99.8% accuracy on all data entry. In a sampling study across our broad user-base, we found the average human makes a mistake on around ~8% of all data fields, or just 92% accuracy. This number varies given time of day and individual energy levels. RaeLM has yet to show signs of a Friday slump, but we’ll report back if this changes.
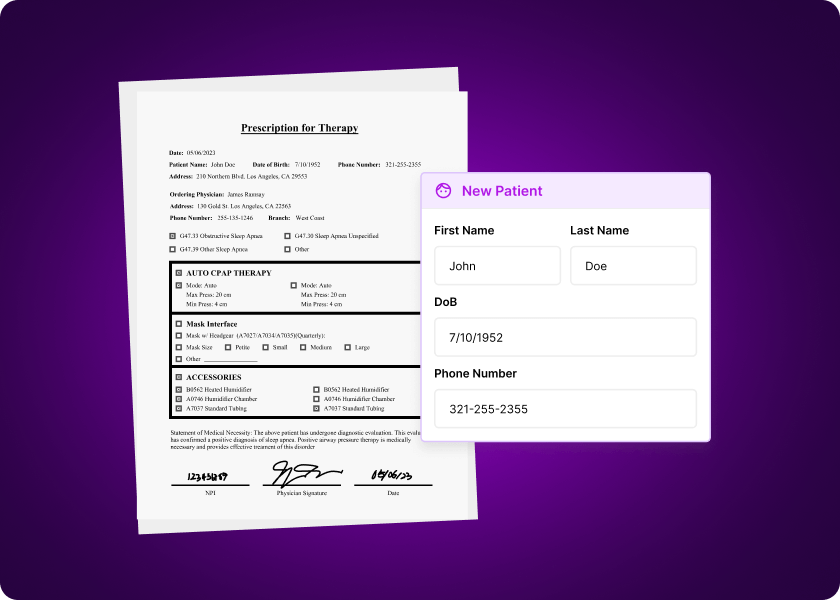
Qualify patients, automatically
Across the tens of thousands of service codes a patient can be treated for (HPPS, HCPCS, CPTs and more) you need to know precisely what documentation must be on hand to get paid and not have revenue clawed back in an audit. Tennr doesn’t just read what’s there, we maintain comprehensive rulesets so that when your documentation is missing we flag it right away. And when it’s all there, make sure you have a ‘proof packet’ for any future audits.
The Machine Learning Under the Hood
Tennr’s Health Code Extractor™ identifies narratives and maps them to a list of codes, from ICDs to HCPCS and CPTs. In many cases, medical staff detail a patient’s needs through shorthand ‘narrative descriptions’, and humans need to then ‘map’ those descriptions to the codes that are expected for successful billing cycles. Knowing what description or what narrative corresponds to what code is what THCE was built to handle. And yes, we call it THCE because we need more confusing acronyms as it relates to decoding medical diagnoses and services.
Our Qualifications Model, (QLM™), uses data extracted by RaeLM and payor guidelines across thousands of CPT & HCPCS codes. QLM assesses the adherence to criteria across all documents pertaining to the patient. This model is actually relatively straightforward and since it takes in an unstructured blob of information and a structured ruleset to be evaluated it is the most-likely to be given the ‘cringe-worthy’ title of true ‘Generative AI’.
Our Qualifications Model, (QLM™), uses data extracted by RaeLM and payor guidelines across thousands of CPT & HCPCS codes. QLM assesses the adherence to criteria across all documents pertaining to the patient. This model is actually relatively straightforward and since it takes in an unstructured blob of information and a structured ruleset to be evaluated it is the most-likely to be given the ‘cringe-worthy’ title of true ‘Generative AI’.

Manage eligibility & benefits from one place
We know which insurance plans you take. You take BCBS, but you don’t take that BCBS. We make sure you have the right details according to nuanced payor guidelines and prior authorizations when needed.
The Machine Learning Under the Hood
Nope, nothing interesting having to do with ML here. Once you’ve tagged all the right pages, split the document by patient and pulled all the right information about the patient including relevant codes, it’s just a matter of running the right eligibility and benefit checks. And we were tired of people taking all that nice clean data from Tennr just to manually run benefit checks and make calls, so we built it right into the platform and an automated add-on. Once you actually know you have the right information for the right plan and payor, there is minimal ‘AI’ at play in actually interacting with the payor via clearinghouse, portal, api, faxing or calling. And you should be skeptical of those claiming there is.

Streamline patient and provider communications
Tennr requests missing information from other providers, keeps referring providers updated with the status of their patients, and keeps patients in the loop.
The Machine Learning Under the Hood
Just like when dealing with insurance, this is not really a machine-learning problem, but rather a systems problem. Since we have all the right information at this point, we know what we’re missing and where we need to go and get it; it’s instead about making sure the right automations are firing to go and get that data. Whether it’s collecting an insurance card from a patient or getting a certificate of medical necessity signed by a referring provider. We’ve configured Tennr to know how to automatically procure that information through a variety of means and stop immediately once the job is done (regardless of whether it came in through a document or a phone call).
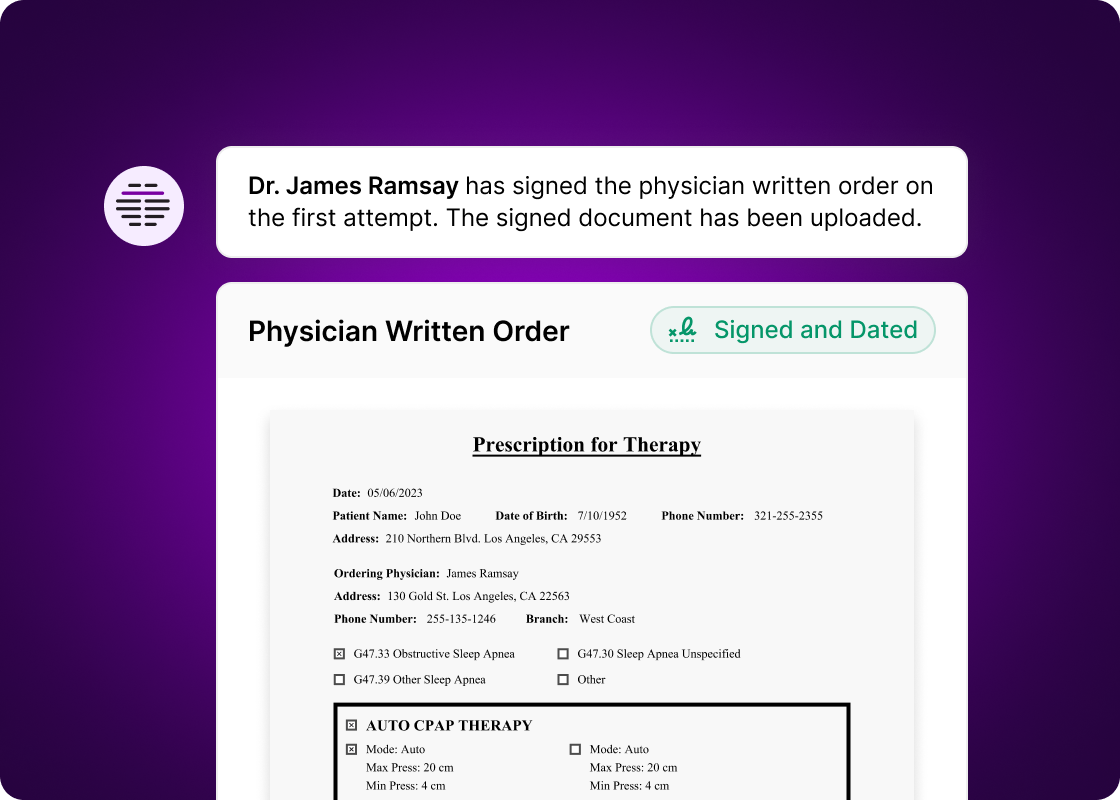
Don't take our word for it
Here's a guy who saw a demo and filmed his product reaction
